Building vs Contracting MLOps Teams: Which Path is Right for Your Business?
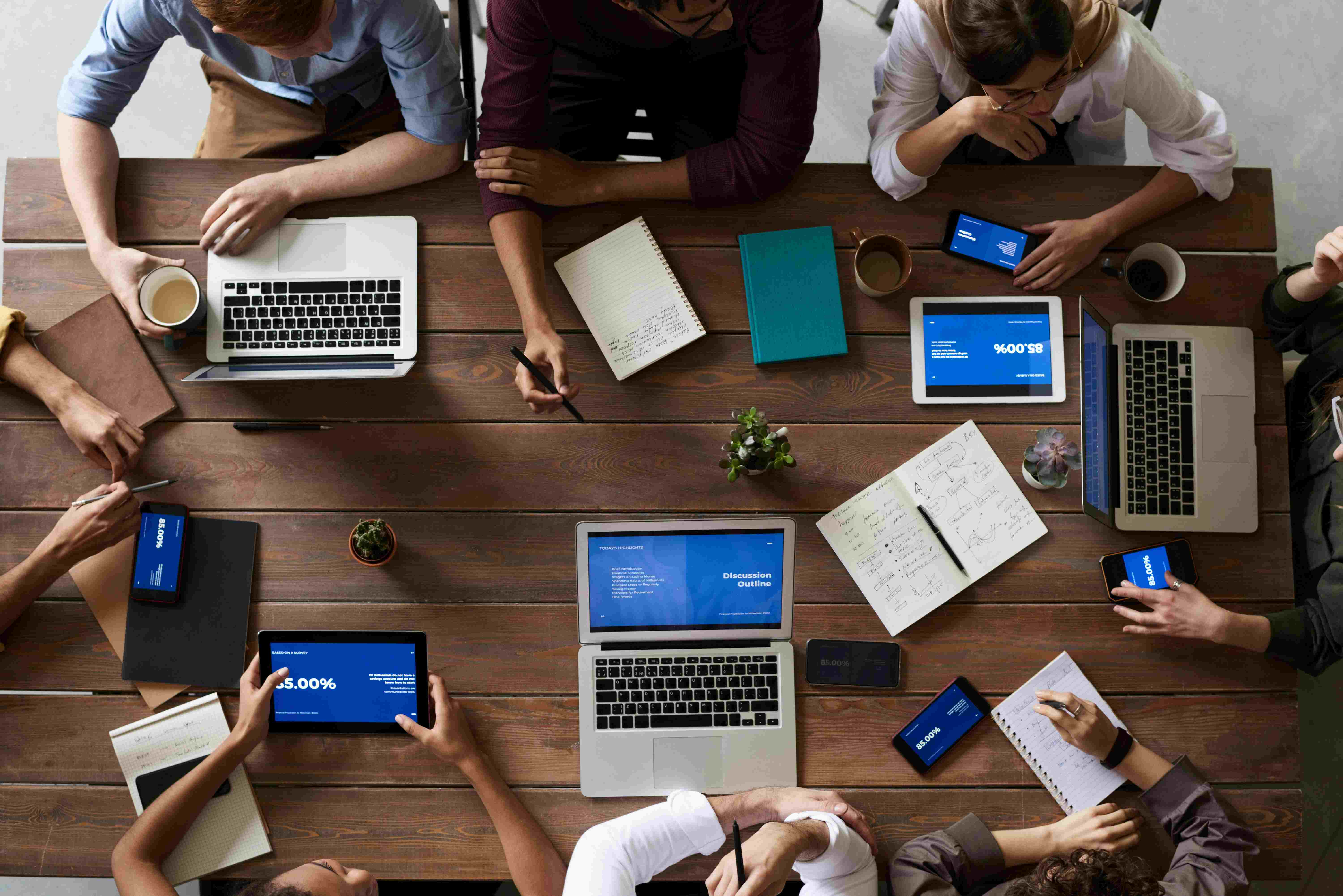
Table of Contents
Introduction
As organizations increasingly rely on data-driven decision making and automation, the implementation of robust MLOps practices has become essential. One of the critical challenges companies face is deciding whether to build an internal MLOps team or to contract external experts for their machine learning operations. In this blog, we break down the benefits and challenges of each approach to help you determine which path aligns best with your business objectives.
Building an In-House MLOps Team
Advantages
1. Deep Integration with Company Culture:
An internal team becomes a seamless part of your organization. They understand your unique workflows, business goals, and can tailor solutions specifically for your needs. This level of integration often leads to faster iteration and long-term process improvements.
2. Enhanced Collaboration and Knowledge Sharing:
In-house teams tend to foster a collaborative environment where data scientists, engineers, and IT professionals work closely together. This cross-functional synergy helps in building production-grade machine learning pipelines and ensures accountability at every stage.
3. Customization and Flexibility:
Having your own team means you can adapt processes and workflows as your business evolves. The continuous exchange of ideas leads to bespoke MLOps practices that can scale with your organization’s growth.
Challenges
1. Talent Acquisition and Retention:
Building a high-performing MLOps team requires significant investment in hiring the right talent. With the field evolving rapidly, finding professionals who have expertise across machine learning, DevOps, and data engineering can be daunting.
2. Higher Upfront Costs:
Investing in recruitment, training, and developing an in-house team may involve substantial upfront costs. This model is typically best suited for organizations with long-term MLOps demands.
3. Time to Operationalize:
Establishing an internal team can be a time-consuming process. The ramp-up period might delay the implementation of critical MLOps practices, impacting short-term project timelines.
Contracting MLOps Experts
Advantages
1. Immediate Expertise and Agility:
Contracting external MLOps experts provides you with immediate access to seasoned professionals who know how to design, implement, and manage MLOps solutions from day one. This can be particularly beneficial if you need to accelerate projects without the overhead of training an internal team.
2. Cost-Efficiency in the Short Term:
For many organizations, especially startups or companies testing the waters with AI, contracting services can be more cost-effective initially. It allows you to pay for expertise on-demand rather than committing to long-term salaries and benefits.
3. Flexibility and Scalability:
Outsourced teams often come with a flexible engagement model. They can scale resources up or down based on project requirements, much like contracting in other industries such as construction where fixed-price or unit-based contracts provide predictability and flexibility.
Challenges
1. Limited Control Over Processes:
While external experts bring in-depth knowledge, they may not be as integrated into your company’s culture or long-term vision. This can sometimes result in solutions that are less tailored to your specific requirements.
2. Potential Communication Gaps:
Contracted teams may operate under different processes and time zones, which can lead to communication challenges. Clear documentation and regular meetings are essential to mitigate these issues.
3. Dependency Risks:
Relying solely on contracted teams could create dependency risks, especially if they hold proprietary knowledge of your systems. Transitioning to an internal team later might then involve steep learning curves.
Key Considerations When Choosing Your Approach
When deciding between building an in-house MLOps team and contracting external experts, consider these factors:
Business Objectives and Timeline: If you need to quickly implement machine learning projects, contracting might be more advantageous. For long-term, strategic growth, investing in an internal team could yield better alignment with your business goals.
Budget and Resource Allocation: Evaluate the upfront costs versus long-term investments. While contracting can be cost-effective in the short term, building a team might reduce recurring costs over time.
Talent Availability: Assess the local talent pool. If expertise in MLOps is scarce in your region, contracting or hybrid models could bridge the gap effectively.
Flexibility vs. Control: Some organizations value the flexibility of external teams, whereas others need full control over every facet of their operations. Understand where your priorities lie.
Conclusion
Choosing between building and contracting MLOps teams is a critical strategic decision. Building an in-house team offers deep integration, customization, and a long-term commitment to your specific needs, but it comes with higher upfront investments and potential delays. On the other hand, contracting external experts delivers rapid deployment, flexibility, and immediate access to experienced professionals, albeit with less control over internal processes.
Ultimately, the choice depends on your organization’s current needs, budget, and long-term vision. Some companies even adopt a hybrid approach: starting with contractual expertise to get projects off the ground, and gradually transitioning to an internal team as their MLOps maturity grows.
By carefully considering these factors, you can tailor your MLOps strategy to drive innovation and operational excellence in your organization.